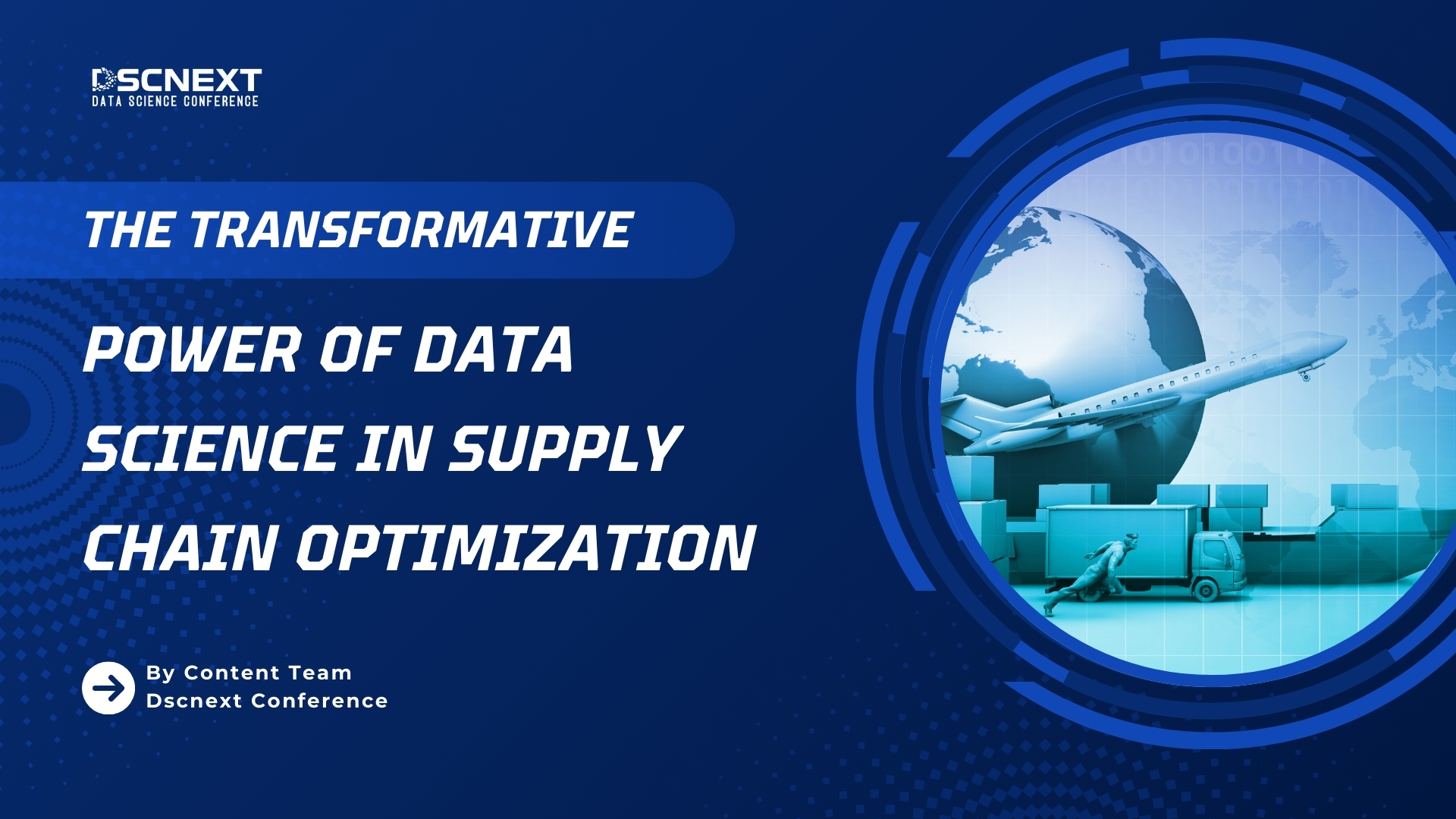
In today’s globalized economy, supply chains have become increasingly intricate, spanning continents and involving multiple stakeholders, from suppliers and manufacturers to distributors and retailers. With this complexity comes a heightened need for optimization—ensuring that each link in the chain functions efficiently to minimize costs, reduce waste, and meet customer demands promptly. This is where data science plays a pivotal role.
Data science, which encompasses statistical analysis, machine learning, and predictive modeling, provides the tools necessary to analyze vast amounts of data, extract actionable insights, and make informed decisions. Data science involves using statistical methods, machine learning algorithms, and predictive analytics to extract meaningful insights from large datasets. In supply chain optimization, these insights can be applied to various aspects such as demand forecasting, inventory management, logistics, and risk mitigation. Key Areas of Impact include:
Demand Forecasting: Predicting customer demand with greater accuracy.
Inventory Management: Balancing stock levels to avoid overstocking or stockouts.
Logistics: Optimizing routes, reducing delivery times, and lowering transportation costs.
Supplier Management: Assessing supplier performance and risks.
Risk Management: Identifying and mitigating potential disruptions in the supply chain.
In this blog, we will delve into the various ways data science is applied to optimize supply chains across different industries, offering a comprehensive understanding of its impact, challenges, and future potential.
1. The Foundation of Supply Chain Efficiency: Demand Forecasting
Demand forecasting is the bedrock upon which successful supply chain management is built. Accurate forecasts enable companies to plan production schedules, manage inventory levels, and optimize logistics. Traditional forecasting methods, which rely heavily on historical sales data and basic statistical techniques, often struggle to account for the myriad factors that can influence demand in today’s fast-paced markets. Walmart, the world’s largest retailer, has turned to data science to enhance its demand forecasting capabilities.
By analyzing an array of data sources—including historical sales, economic indicators, weather forecasts, and even social media activity—Walmart’s data scientists can predict demand for specific products with a high degree of accuracy. This allows the company to adjust its inventory levels dynamically, ensuring that popular items are always available while reducing the risk of overstocking less popular products. In the food industry, where product freshness is critical, accurate demand forecasting can significantly impact a company’s bottom line. Danone, a global leader in dairy and plant-based products, uses data science to forecast demand across its product lines.
By integrating data from point-of-sale systems, social media sentiment analysis, and market research, Danone can anticipate shifts in consumer preferences and adjust production accordingly. This not only reduces waste but also ensures that the freshest products reach consumers.
2. Inventory Management: Balancing Cost and Availability
Inventory management is a critical component of supply chain optimization. Companies must strike a delicate balance between holding enough stock to meet customer demand and minimizing the costs associated with excess inventory. Data science provides the tools to achieve this balance by analyzing demand patterns, lead times, and supply chain constraints. Toyota, renowned for its lean manufacturing principles, applies data science to optimize inventory management across its global operations.
By utilizing machine learning algorithms to analyze production data, sales forecasts, and supply chain variables, Toyota can predict the demand for specific car models and their components. This allows the company to maintain a just-in-time (JIT) inventory system, where parts are delivered to the assembly line exactly when needed, minimizing storage costs and reducing waste.
In the healthcare industry, where timely availability of medical supplies can be a matter of life and death, efficient inventory management is crucial. Johnson & Johnson, a leading healthcare company, uses data science to ensure that hospitals and clinics have access to critical medical supplies when they need them. By analyzing data on hospital admissions, patient treatment patterns, and supplier lead times, Johnson & Johnson can forecast demand for items like surgical instruments, pharmaceuticals, and medical devices, ensuring optimal inventory levels and reducing the risk of shortages.
3. Logistics and Transportation: Optimizing the Flow of Goods
Logistics and transportation are among the most complex and costly components of the supply chain. Data science offers powerful tools to optimize these processes, reducing transportation costs, improving delivery times, and enhancing overall efficiency. By analyzing data on traffic patterns, fuel prices, vehicle maintenance, and delivery schedules, companies can make data-driven decisions to streamline their logistics operations. Amazon’s success as the world’s largest e-commerce platform is largely due to its ability to deliver products to customers quickly and efficiently. Data science is at the heart of Amazon’s logistics strategy.
The company employs machine learning models to analyze real-time data from GPS, weather forecasts, traffic conditions, and customer orders. This allows Amazon to optimize delivery routes, allocate resources effectively, and predict package delivery times with remarkable accuracy. The result is a logistics network that not only reduces fuel consumption and operational costs but also meets customer expectations for rapid delivery. In the dairy industry, where products are perishable, efficient logistics are essential to ensure that fresh goods reach consumers on time. Amul, one of India’s largest dairy cooperatives, leverages data science to optimize its extensive supply chain.
With thousands of collection centers, processing plants, and distribution points, Amul uses predictive analytics to determine the most efficient routes for transporting raw milk and dairy products. Factors such as temperature control, distance, and delivery times are analyzed to minimize spoilage and ensure that products arrive at retail outlets in peak condition.
4. Supplier Management and Risk Mitigation
Managing a global network of suppliers involves numerous challenges, including quality control, delivery reliability, and the risk of disruptions due to geopolitical events, natural disasters, or economic shifts. Data science equips companies with the tools to monitor supplier performance, predict potential risks, and develop contingency plans to mitigate these risks. Apple’s supply chain is one of the most complex in the world, involving thousands of suppliers across multiple countries. To manage this complexity, Apple uses data science to monitor and assess supplier performance in real-time.
By analyzing data on production quality, delivery times, and geopolitical risks, Apple can identify potential issues before they escalate into major disruptions. This proactive approach allows Apple to make strategic decisions, such as diversifying its supplier base or adjusting production schedules, ensuring that product launches and deliveries occur on time. Zara, the fast-fashion giant, is known for its ability to rapidly bring new designs to market. This agility is supported by a highly optimized supply chain, where data science plays a critical role.
Zara uses predictive analytics to manage its network of suppliers and manufacturers, analyzing data on fabric availability, production lead times, and transportation costs. By continuously monitoring these factors, Zara can quickly adapt to changing fashion trends, producing and distributing new collections in a matter of weeks rather than months. This responsiveness gives Zara a significant competitive edge in the fast-paced fashion industry.
5. The Challenges of Implementing Data Science in Supply Chains
While the benefits of data science in supply chain optimization are clear, implementing these technologies is not without challenges. Companies must navigate issues related to data quality, system integration, and the skills gap in the workforce.
Data Quality: High-quality data is essential for accurate analysis and decision-making. However, supply chains often involve multiple stakeholders, each using different systems and formats for data collection. Ensuring data consistency and accuracy across these disparate sources is a significant challenge that companies must address through robust data governance practices.
System Integration: Supply chains are complex, involving various systems for procurement, production, inventory management, and logistics. Integrating these systems to create a seamless flow of data is crucial for successful optimization. Advanced data integration tools, along with cloud-based platforms, are increasingly being used to overcome these challenges, enabling companies to harness the full potential of their data.
Skills Gap: The growing demand for data scientists and analytics professionals has created a skills gap in the market. Companies must invest in training and development to build in-house expertise, or they risk falling behind in their ability to leverage data science for supply chain optimization. Collaborative partnerships with academic institutions and specialized training programs can help address this gap.
6. The Future of Data-Driven Supply Chains
The future of supply chain optimization lies in the continued advancement of data science and related technologies. Artificial intelligence (AI), the Internet of Things (IoT), and blockchain are poised to further revolutionize supply chain management, enabling even greater levels of efficiency, transparency, and resilience.
AI-Powered Automation: As AI technologies mature, we can expect to see more widespread adoption of automated decision-making processes in supply chains. AI-driven systems can analyze vast amounts of data in real-time, identifying patterns and making decisions faster and more accurately than human operators. This will enable supply chains to respond more dynamically to changes in demand, disruptions, or other unforeseen events.
IoT and Real-Time Analytics: The integration of IoT devices into supply chains will provide unprecedented levels of visibility and control. Sensors embedded in products, vehicles, and warehouses will continuously transmit data, allowing for real-time monitoring of inventory levels, shipment conditions, and equipment status. Coupled with advanced analytics, this data will enable companies to optimize their supply chains with a level of precision that was previously unattainable.
Blockchain for Transparency and Security: Blockchain technology offers the potential to enhance transparency and security in supply chains. By creating an immutable ledger of transactions, blockchain can provide a verifiable record of every step in the supply chain, from raw material sourcing to final delivery. This transparency can help companies ensure the integrity of their products, reduce the risk of fraud, and build trust with consumers.
Data science is transforming supply chain management, providing companies with the tools they need to navigate the complexities of a globalized economy. From demand forecasting and inventory management to logistics optimization and supplier risk mitigation, data-driven insights are helping businesses enhance efficiency, reduce costs, and improve customer satisfaction.
As technology continues to evolve, the role of data science in supply chain optimization will only grow more critical. Companies that invest in data science today will be better positioned to thrive in the increasingly competitive and dynamic markets of the future. As data science continues to evolve, its impact on supply chain management will only grow, making it an essential tool for businesses aiming to stay competitive in a rapidly changing global market.
The future of data science is bright, with AI at its core, driving the next wave of technological innovation. Companies that invest in data science will be better positioned to navigate the complexities of the modern world, make informed decisions, and achieve long-term success. Embark on a groundbreaking journey at the inaugural Data Science Next Conference May 7-9 2025, in Amsterdam by NBM, where pioneers and visionaries will gather to chart new territories in data science.
As a debut event, this conference offers an unparalleled opportunity to be among the first to explore fresh perspectives, engage with cutting-edge methodologies, and contribute to shaping the future of the field. Designed for those eager to push boundaries and spark innovation, this event promises to ignite your curiosity and provide the foundational insights needed to navigate the evolving landscape of data science. Join us as we set the stage for the next era of data-driven innovation.