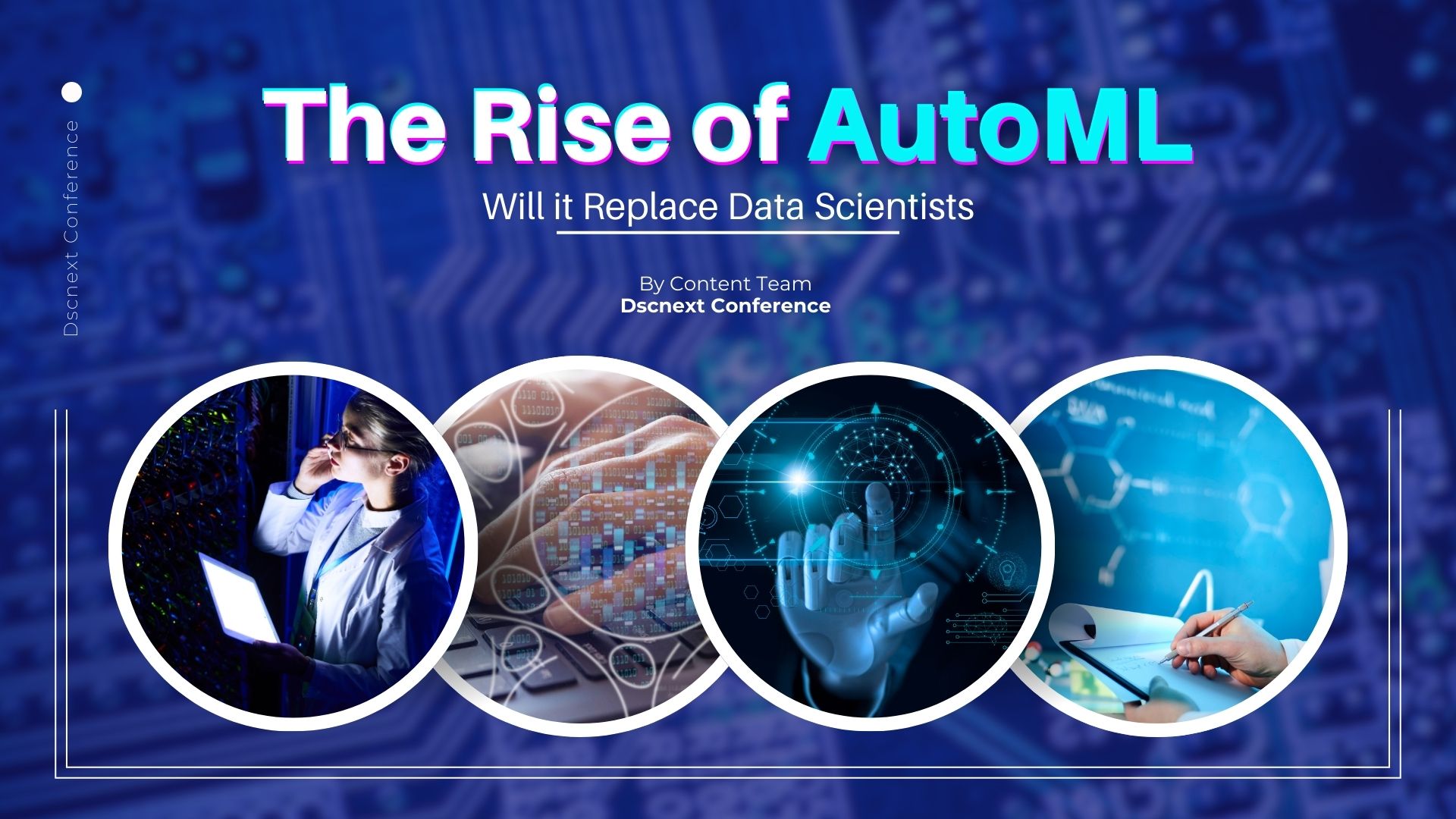
The rise of Automated Machine Learning (AutoML) has sparked considerable debate in the tech world. AutoML, a tool designed to automate the end-to-end process of applying machine learning to real-world problems, promises to democratize data science by making complex algorithms accessible to non-experts. While AutoML tools are undeniably powerful, many wonder: Will AutoML replace data scientists, or will it become another tool in their arsenal?
In this blog, we’ll explore the growing role of AutoML, its benefits, limitations, and whether it poses a real threat to data science professionals.
What Is AutoML?
AutoML refers to platforms and tools that automate the machine learning workflow, including data preprocessing, feature engineering, model selection, hyperparameter tuning, and model evaluation. It reduces the need for manual intervention, allowing users with limited machine learning expertise to build robust models.
Key components of AutoML include:
- Data Preprocessing: Automatically cleaning and preparing raw data for analysis.
- Feature Engineering: Automatically identifying relevant features in a dataset.
- Model Selection: Automating the selection of the most appropriate algorithm for a given problem.
- Hyperparameter Tuning: Automating the optimization of model parameters for better performance.
- Model Evaluation: Automatically assessing model performance and suggesting improvements.
Popular AutoML platforms like Google Cloud AutoML, H2O.ai, and Microsoft Azure AutoML have made it possible for businesses to deploy machine learning models faster, even without specialized data science teams.
Benefits of AutoML
- Accessibility for Non-Experts AutoML opens the doors of machine learning to non-experts, enabling business analysts, developers, and engineers to build models without needing in-depth knowledge of algorithms or coding. For organizations with limited data science resources, this is a huge advantage.
- Speed and Efficiency With automation at its core, AutoML significantly speeds up the model development process. Tasks that would typically take weeks or months for a data science team can be completed in a matter of hours or days. This accelerated workflow is critical in industries where quick decision-making is essential.
- Cost Reduction By reducing the dependency on highly specialized data scientists, AutoML can lower the overall costs of machine learning projects. Smaller companies or startups that may not have the budget to hire full-time data scientists can still benefit from machine learning insights.
- Automated Hyperparameter Tuning Hyperparameter tuning is a time-consuming aspect of model development. AutoML excels in automating this process, helping to identify the most optimized model configurations without trial and error.
Limitations of AutoML
While AutoML has its advantages, it’s far from perfect and comes with some key limitations.
- Lack of Domain Expertise AutoML may automate technical processes, but it lacks the deep understanding of domain-specific knowledge that data scientists bring. Data scientists play a critical role in interpreting results, understanding context, and identifying business-specific insights that AutoML tools can miss.
- Black Box Models One of the main criticisms of AutoML is the lack of interpretability in the models it generates. While AutoML tools can produce high-performing models, they often act as “black boxes,” making it difficult to understand how decisions are being made. In industries like healthcare and finance, where explainability is essential, this can be a significant limitation.
- Customization Limitations AutoML platforms are not as flexible as a skilled data scientist. Complex, custom solutions—particularly those requiring novel approaches, advanced algorithms, or specialized data—may be beyond the capabilities of AutoML. In these cases, human expertise is still needed to design, tweak, and improve models.
- Data Quality Challenges AutoML can struggle with poor or incomplete datasets. While data preprocessing is automated, the quality and structure of the data still matter, and AutoML tools may not handle data-related challenges as effectively as human data scientists.
Will AutoML Replace Data Scientists?
The short answer is no, AutoML will not replace data scientists—but it will change how they work. Rather than eliminating data science roles, AutoML will likely complement them by handling repetitive tasks, freeing up data scientists to focus on more complex and creative aspects of their work.
Here’s why:
- Complex Problem Solving: AutoML is excellent for automating routine tasks, but data scientists bring critical thinking, problem-solving skills, and creativity to the table. Many machine learning projects require custom models, unique feature engineering, and domain-specific insights that cannot be automated.
- Interpretation and Storytelling: Data science is not just about building models. It’s about interpreting the results, understanding the business context, and communicating findings in a way that decision-makers can understand. AutoML can generate models, but it cannot tell the story behind the data.
- Innovative Approaches: While AutoML automates existing algorithms, data scientists push the boundaries of machine learning by developing new models, testing novel approaches, and improving current methodologies. This level of innovation cannot be replaced by automation.
- Ethical and Responsible AI: Data scientists are increasingly responsible for ensuring that AI models are ethical, unbiased, and used responsibly. AutoML tools lack the human judgment needed to consider the broader societal implications of AI, making human oversight essential.
The Future of Data Science with AutoML
Instead of viewing AutoML as a threat, data scientists can see it as a tool that enhances their capabilities. By automating routine tasks, AutoML allows data scientists to focus on more valuable activities, such as strategic decision-making, advanced analytics, and innovation. It also provides a way for non-experts to experiment with machine learning, making AI more accessible across organizations.
As AutoML continues to evolve, we will likely see more collaboration between automated tools and human expertise. AutoML will not replace data scientists, but it will redefine their roles, leading to a more efficient and productive future for the data science field.
Conclusion: A Collaborative Future
The rise of AutoML marks an exciting phase in the world of data science. While it brings automation and efficiency, it is not a replacement for human expertise. Data scientists will continue to play a crucial role in building, refining, and interpreting models—especially in complex, high-stakes projects. The future lies in collaboration, where AutoML enhances the capabilities of data scientists, rather than replacing them.
Are you ready to embrace the future of data science with AutoML? By understanding its strengths and limitations, organizations can leverage AutoML as a powerful tool to complement human expertise, unlocking new possibilities in the world of machine learning.