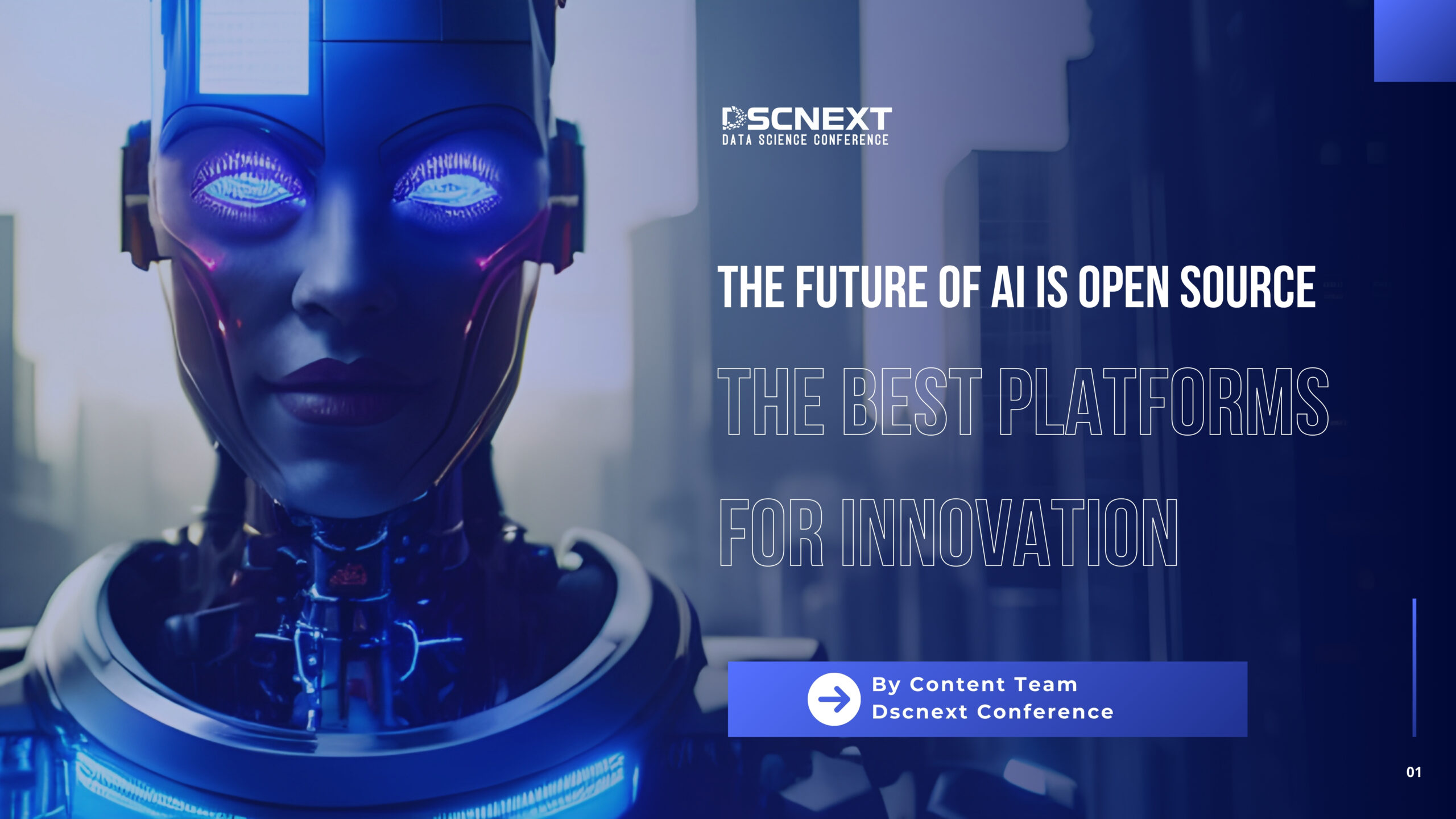
Artificial Intelligence (AI) has become an integral part of modern life, with applications spanning from personalized recommendations to complex medical diagnostics. At the heart of this technological revolution lies *open-source AI*, a movement that makes AI tools and platforms accessible to anyone with the skills to use them.
Developers, researchers, and businesses alike are turning to open-source AI to drive innovation and transform industries. In this blog, we’ll explore what open-source AI is, its advantages and challenges, and take a closer look at some of the top platforms fueling this global trend.
What is Open-Source AI?
Open-source AI refers to AI tools, frameworks, and libraries that are freely accessible for anyone to use, modify, and distribute. Unlike proprietary AI systems, open-source AI enables collaboration across a global community of developers, leading to the rapid advancement of innovative solutions.
These projects are hosted on platforms like GitHub, and their open nature allows small development teams, educational institutions, and even large enterprises to benefit from cutting-edge AI technologies without being locked into costly vendor solutions.
Open-source AI promotes transparency, fosters collaboration, and democratizes access to powerful AI tools, driving significant progress in fields like healthcare, finance, education, and more. By utilizing existing libraries and frameworks, developers can save time and focus on crafting tailored solutions to real-world challenges.
Advantages of Open-Source AI
The open-source approach to AI comes with several key benefits that make it a compelling choice for developers, researchers, and organizations
1. Diverse Use Cases: From real-time fraud detection to medical image analysis, open-source AI platforms provide solutions for a wide array of applications. This versatility helps drive innovation across industries.
2. Accessibility: Open-source AI is available to anyone, offering an inclusive platform for learning, experimentation, and innovation. It levels the playing field, allowing even small teams to access state-of-the-art tools.
3. Community Engagement: Open-source projects thrive on community collaboration. Developers worldwide contribute to improving and expanding these tools, ensuring that they stay relevant and up-to-date.
4. Transparency and Improvement: The open nature of these platforms encourages continuous improvement. Issues like bugs or security concerns are more likely to be addressed quickly by the community, leading to more dependable and feature-rich solutions.
5. Vendor Neutrality: Open-source AI frees organizations from being tied to any one provider, offering flexibility in technology choices and reducing long-term costs.
Challenges of Open-Source AI
Despite its numerous benefits, open-source AI also comes with its own set of challenges:
1. Risk of Misalignment: Without clear goals, organizations can struggle to leverage open-source AI effectively, potentially leading to misaligned outcomes or project failure.
2. Algorithmic Bias: Open-source AI algorithms may inherit biases from the data they are trained on, which could lead to flawed results and unintended consequences.
3. Security Concerns: Open access to AI tools increases the risk of malicious actors exploiting them for harmful purposes.
4. Data Management Issues: Poor quality training data can result in ineffective models, while data drift and labeling errors can make AI models unreliable.
5. Outsourced Technology Risks: Using open-source AI developed by external entities could expose an organization to risks, including intellectual property issues or lack of support.
Top 10 Open-Source AI Platforms Driving Innovation
The rise of open-source AI has led to the creation of numerous platforms designed to meet diverse needs. Below are 10 of the most popular and widely adopted platforms in the industry today:
1. TensorFlow: A comprehensive machine learning framework developed by Google, TensorFlow supports multiple languages such as Python and JavaScript, allowing developers to build and deploy models across various devices. Its strength lies in its flexibility and scalability, though beginners may find it complex due to its lower-level API.
2. PyTorch: Known for its intuitive interface and dynamic computation graphs, PyTorch simplifies deep learning model development. Researchers favor PyTorch for its quick prototyping capabilities, especially in computer vision and natural language processing. It’s easy to debug but might be less performant than TensorFlow for extremely large models.
3. Keras: Sitting atop libraries like TensorFlow and PyTorch, Keras is renowned for its user-friendly high-level API. It enables swift prototyping of deep learning models, making it a popular choice for beginners and advanced users alike. Its ease of use comes at the expense of lower-level control, but it’s ideal for those looking to develop deep learning applications quickly.
4. OpenAI: OpenAI, the research company behind GPT models, has made significant strides in advancing reinforcement learning and natural language processing (NLP). Tools like OpenAI Gym are popular for reinforcement learning research, while their GPT models are used widely for NLP tasks. OpenAI tools are cutting-edge but may lack the polish of more established platforms.
5. Rasa: This open-source conversational AI platform is designed for creating chatbots and virtual assistants. With its flexible architecture, Rasa allows developers to customize their conversational agents, making it ideal for complex, business-specific use cases.
6. Amazon SageMaker: While not purely open-source, SageMaker integrates with many open-source tools and simplifies building, training, and deploying machine learning models at scale. As part of Amazon Web Services (AWS), it offers robust scalability but could lock users into the AWS ecosystem.
7. Apache MXNet: Known for its flexibility and efficiency, MXNet combines symbolic and imperative programming modes, making it suitable for both research and production. It’s highly scalable but might have a steeper learning curve than more user-friendly platforms.
8. Scikit-learn: A widely-used machine learning library in Python, Scikit-learn offers tools for data mining and predictive analysis. It’s ideal for classical machine learning tasks and integrates seamlessly with other Python libraries like Pandas and NumPy.
9. OpenCV: This library focuses on computer vision tasks like object detection, image processing, and video analysis. OpenCV is highly performant and real-time capable, making it popular in industries needing image-based automation.
10. H2O.ai: Offering a wide range of algorithms for tasks like data preprocessing and model selection, H2O.ai is a versatile platform for building machine learning models. Its automated tools and user-friendly interface make it accessible, though the free version has limitations compared to the enterprise-grade option.
The Future of Open-Source AI
The future of open-source AI is promising. With advancements in areas like natural language processing and computer vision, platforms like Hugging Face Transformers and OpenCV are enabling the creation of more sophisticated applications, from chatbots to automated visual inspections.
However, organizations adopting open-source AI need to invest in expertise to customize and fine-tune these tools for enterprise use, ensuring trust and reliability. Open-source AI will continue to democratize access to cutting-edge technologies, but careful implementation and strong partnerships are essential to realizing its full potential. The right balance between open-source flexibility and tailored AI solutions could drive meaningful transformation across industries for years to come.
By leveraging these open-source AI platforms, developers and organizations can unlock new possibilities and drive innovation across industries, making AI more accessible and effective than ever before.Companies that invest in data science will be better positioned to navigate the complexities of the modern world, make informed decisions, and achieve long-term success. Embark on a groundbreaking journey at the inaugural Data Science Next Conference May 7-9 2025, in Amsterdam by NBM, where pioneers and visionaries will gather to chart new territories in data science.
As a debut event, this conference offers an unparalleled opportunity to be among the first to explore fresh perspectives, engage with cutting-edge methodologies, and contribute to shaping the future of the field. Designed for those eager to push boundaries and spark innovation, this event promises to ignite your curiosity and provide the foundational insights needed to navigate the evolving landscape of data science. Join us as we set the stage for the next era of data-driven innovation.