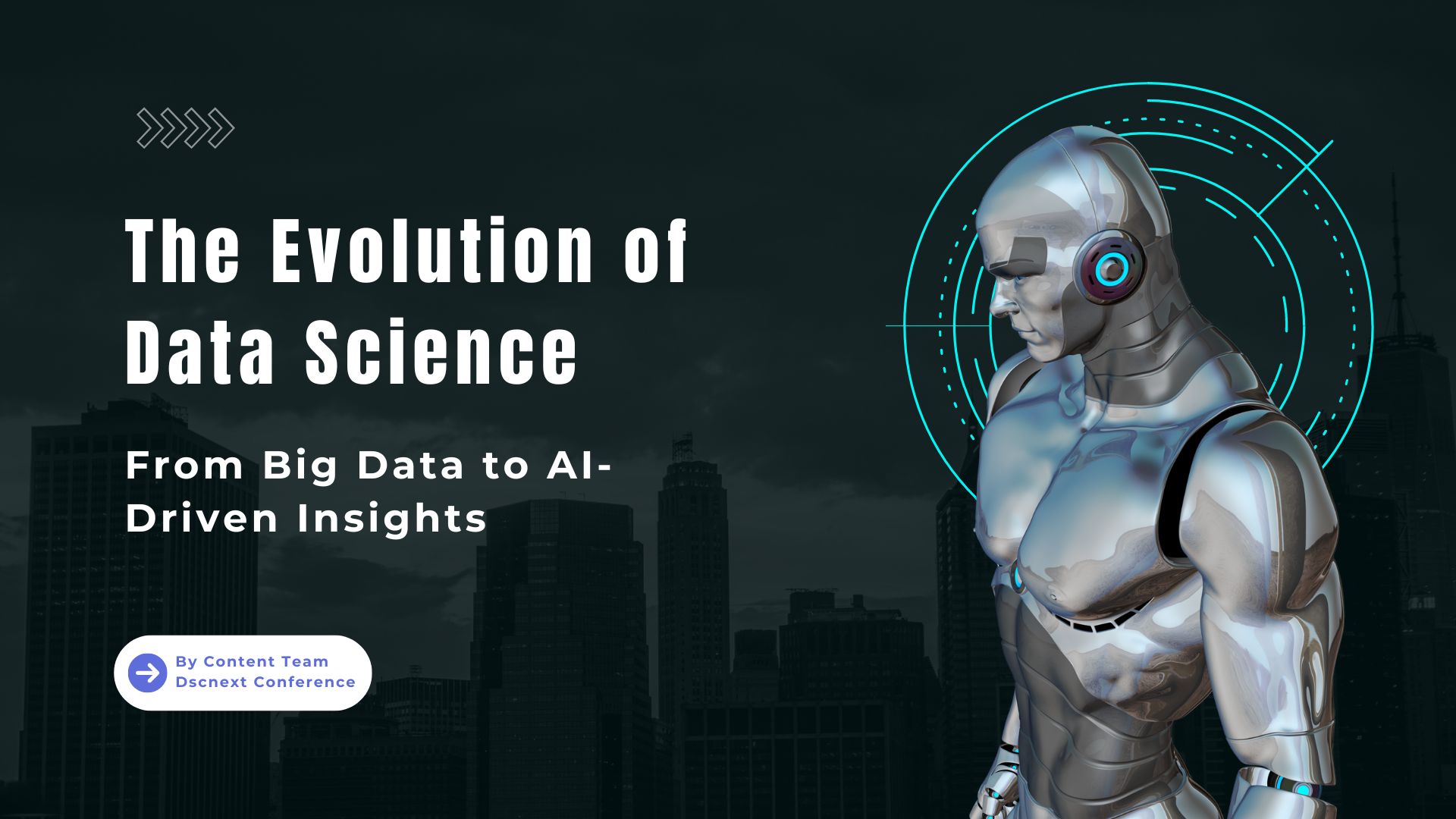
Data science has undergone a transformative journey, evolving from the era of Big Data to the age of AI-driven insights. This evolution has reshaped industries, enhanced decision-making processes, and opened up new avenues for innovation. In this blog, we will explore this journey in detail, examining how the field has evolved and the real-life applications that showcase its impact.
The Big Data Era: Laying the Groundwork
The Big Data era was marked by the explosion of data generated by digital sources, leading to challenges in storing, processing, and analyzing these vast datasets. The focus during this period was on developing technologies that could handle the “3 Vs”—Volume, Variety, and Velocity. Key technologies like Hadoop and Apache Spark were developed to manage and process large-scale data, enabling organizations to harness the power of Big Data for business insights.
Walmart utilized Big Data to optimize its supply chain, analyzing vast amounts of sales data to predict demand and manage inventory more efficiently. This data-driven approach helped Walmart reduce costs, minimize stockouts, and improve customer satisfaction.
The Emergence of Data Science: Transforming Data into Insights
Data science emerged as a field focused on extracting actionable insights from data. This shift was driven by the need to go beyond data management and utilize data for strategic decision-making. The development of programming languages like Python and machine learning libraries like TensorFlow allowed data scientists to build predictive models and uncover patterns that informed business strategies.
Netflix revolutionized content consumption by using data science to develop its recommendation engine. By analyzing user behavior, Netflix could suggest content tailored to individual preferences, significantly improving user engagement and retention.
The Rise of Machine Learning: Enhancing Predictive Analytics
Machine learning (ML) is a subset of data science that focuses on developing algorithms capable of learning from data and making predictions or decisions. ML enabled organizations to automate predictive analytics and develop more sophisticated models.
ML shifted the focus from descriptive and diagnostic analytics towards predictive analytics, allowing businesses to anticipate trends and behaviors with greater accuracy. Amazon uses machine learning to optimize pricing in real time. By analyzing customer behavior, market trends, and competitor prices, Amazon’s ML algorithms dynamically adjust prices, ensuring both competitiveness and profitability.
The AI-Driven Insights Era: The New Frontier
The integration of Artificial Intelligence (AI) into data science has revolutionized how insights are derived from data. AI-driven insights leverage advanced algorithms, including deep learning and neural networks, to analyze data and uncover complex patterns that were previously undetectable.
AI’s Impact on Industries
AI-driven insights have transformed various sectors by enabling more accurate predictions, enhanced decision-making, and automation of complex tasks. PathAI is a pioneer in using AI-driven insights to improve diagnostic accuracy in healthcare. By analyzing pathology images, PathAI’s AI algorithms can detect cancerous tissues with greater precision than traditional methods, leading to earlier diagnosis and better patient outcomes.
In the finance sector, companies like PayPal and Mastercard use AI-driven insights to enhance fraud detection. AI models analyze transaction data in real-time to identify suspicious activities, reducing fraud rates and improving customer trust. For instance, Mastercard’s AI system analyzes over 75 billion transactions annually, detecting fraudulent patterns with remarkable accuracy.
AI-driven insights are also revolutionizing agriculture through precision farming. Companies like John Deere use AI to analyze data from sensors, drones, and satellite imagery to optimize crop yields, reduce water usage, and improve overall farm efficiency.
By predicting weather patterns, soil conditions, and crop health, AI helps farmers make more informed decisions, leading to higher productivity and sustainability. In retail, companies like H&M and Zara use AI-driven insights to personalize the shopping experience.
By analyzing customer data, these retailers can tailor recommendations, optimize inventory management, and create targeted marketing campaigns. AI algorithms analyze purchase history, browsing behavior, and even social media interactions to predict customer preferences and trends.
AI-driven insights are playing a crucial role in the energy sector, particularly in optimizing grid management. Companies like Siemens and GE use AI to analyze data from smart grids, predicting energy demand and optimizing the distribution of electricity. This not only improves efficiency but also reduces costs and minimizes the environmental impact of energy consumption.
Latest Developments in AI-Driven Data Science
Generative AI and Large Language Models
The development of generative AI models, like OpenAI’s GPT-4, represents a significant advancement in AI-driven data science. These models can generate human-like text, create content, and even assist in complex decision-making processes across various industries. For instance, GPT-4 is being used in customer service automation, content creation, and legal document drafting, enhancing efficiency and reducing costs.
Multimodal AI
Multimodal AI is another cutting-edge development, combining different types of data (text, images, audio) to provide more comprehensive insights. This approach is being used in fields like autonomous driving, where AI systems analyze visual, auditory, and sensor data to make real-time decisions.
Ethical AI and Explainable AI (XAI)
As AI systems become more integrated into decision-making processes, the demand for ethical AI and explainable AI (XAI) is growing. XAI focuses on making AI’s decision-making process transparent and understandable to humans, ensuring fairness and accountability. For example, in healthcare, XAI is used to ensure that AI-driven diagnostic tools are transparent and do not introduce biases in treatment recommendations.
AI in Drug Discovery
In drug discovery, AI-driven insights are accelerating the development of new treatments. Companies like Insilico Medicine and BenevolentAI use AI to analyze biological data and predict the efficacy of drug candidates. This approach reduces the time and cost associated with bringing new drugs to market, potentially saving lives by speeding up the availability of critical treatments.
The Future of Data Science: AI and Beyond
The future of data science will be shaped by the convergence of AI with other emerging technologies, such as quantum computing, the Internet of Things (IoT), and blockchain. Quantum computing, in particular, holds the potential to revolutionize data processing speeds, enabling the analysis of even more complex datasets.
As AI becomes more pervasive, the ethical implications of AI systems will become increasingly important. The development of AI governance frameworks will be crucial in ensuring that AI is used responsibly and that its benefits are widely shared.
The European Union’s AI Act is one of the first major regulatory efforts to address these concerns, setting standards for AI transparency, fairness, and accountability. Google DeepMind continues to push the boundaries of AI research, with recent breakthroughs in areas like protein folding and energy efficiency.
DeepMind’s AI was used to predict the 3D structure of proteins, a task critical to drug discovery, which could lead to the development of new treatments for diseases. Additionally, DeepMind’s AI has been applied to optimize energy consumption in data centers, significantly reducing their carbon footprint.
The evolution of data science from Big Data to AI-driven insights has been a journey of innovation and transformation. AI-driven insights have unlocked new possibilities for industries, enabling more accurate predictions, enhanced decision-making, and automation of complex tasks.
As AI continues to advance, the integration of emerging technologies will further expand the possibilities of data science, offering new opportunities and challenges for businesses and society. The future of data science is bright, with AI at its core, driving the next wave of technological innovation.
Companies that invest in data science will be better positioned to navigate the complexities of the modern world, make informed decisions, and achieve long-term success. Embark on a groundbreaking journey at the inaugural Data Science Next Conference May 7-9 2025, in Amsterdam by NBM, where pioneers and visionaries will gather to chart new territories in data science.
As a debut event, this conference offers an unparalleled opportunity to be among the first to explore fresh perspectives, engage with cutting-edge methodologies, and contribute to shaping the future of the field. Designed for those eager to push boundaries and spark innovation, this event promises to ignite your curiosity and provide the foundational insights needed to navigate the evolving landscape of data science. Join us as we set the stage for the next era of data-driven innovation.