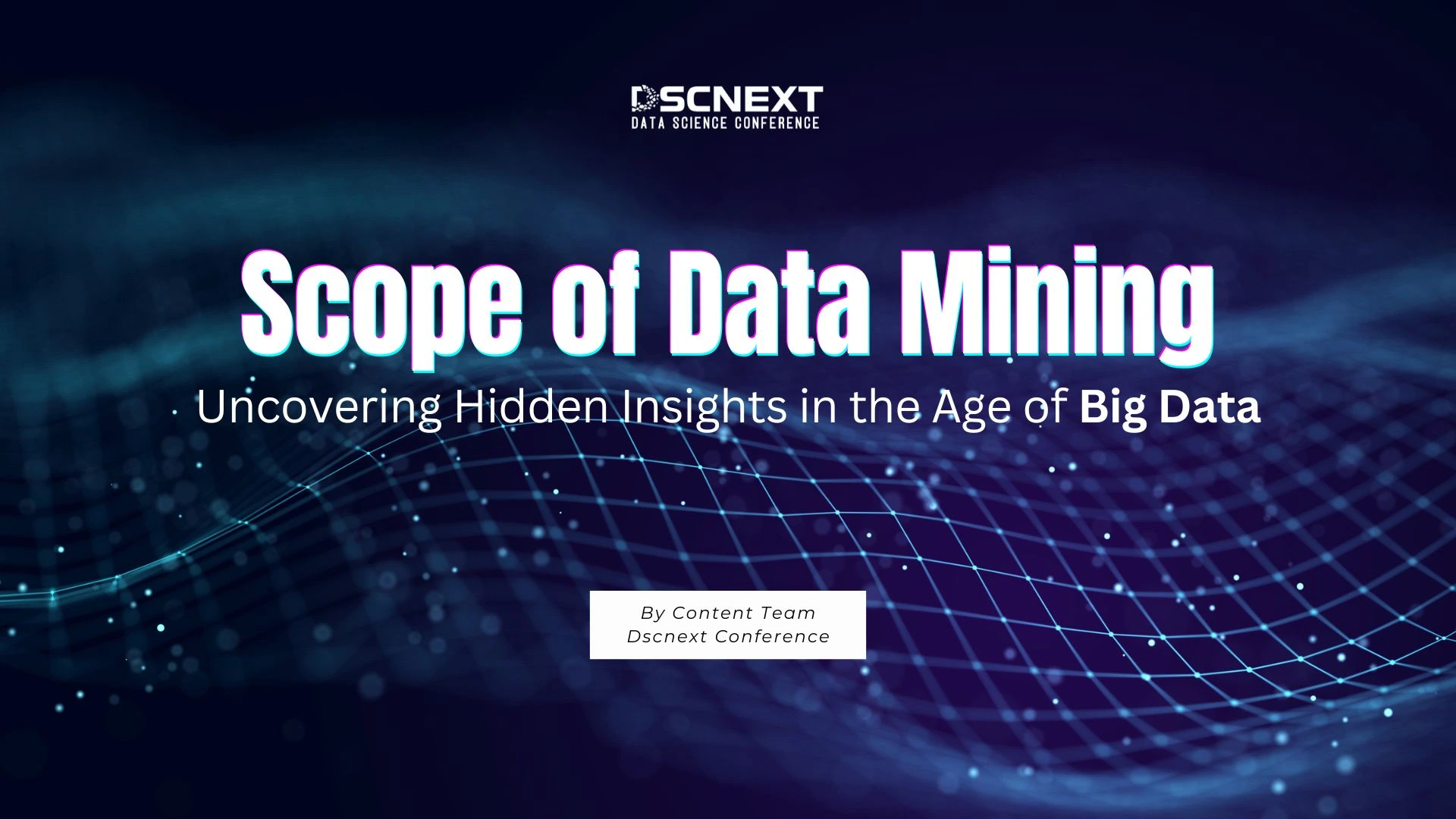
Introduction
The Data Explosion
In today’s data-driven world, Organizations are inundated with vast amounts of data but struggle to extract meaningful insights
that can drive business success. With the exponential growth of big data, the amount of information generated every day is staggering—over 2.5 quintillion bytes of data are created daily, equivalent to 500 million hours of Netflix streaming.
With this overwhelming influx of data, businesses require efficient methods to extract valuable insights, make informed decisions, and stay ahead of the competition. This is where data mining becomes an essential tool.
What is Data Mining?
Data mining is the use of machine learning and statistical analysis to uncover patterns and valuable information from large datasets. Also known as knowledge discovery in databases (KDD), data mining has accelerated over the past decade due to advancements in:
Machine Learning (ML): Automates model training for accurate predictions.
Data Warehousing: Enables centralized storage for large-scale analysis.
Big Data Technologies: Tools like Apache Spark facilitate fast processing.
Despite its rapid evolution, challenges such as scalability and automation persist for organizations looking to leverage data mining effectively.
How Data Mining Works
Data mining techniques can be deployed for two main purposes:
1. Descriptive Analysis – Identifying patterns, trends, and correlations in data.
2. Predictive Analysis – Using machine learning algorithms to forecast future outcomes.
These methods help businesses organize and filter data, surfacing the most useful insights—ranging from fraud detection and user behavior analysis to security breach identification. When combined with AI-powered automation, data mining accelerates decision-making.
Furthermore, data analytics and visualization tools like Apache Spark make it easier than ever to extract meaningful insights efficiently.
Applications of Data Mining Across Industries
Data mining is reshaping industries by offering predictive insights and automation.
Finance – Fraud detection, risk assessment, stock market predictions.
Healthcare – Disease prediction, patient monitoring, drug discovery.
Retail & E-commerce – Customer segmentation, recommendation systems, inventory management.
Cybersecurity – Identifying security breaches, detecting anomalies.
Agriculture – Precision farming, yield prediction, soil health analysis.
Real-world examples of successful data mining applications:
Walmart’s Supply Chain Optimization: Data-Driven Efficiency
Walmart, one of the world’s largest retailers, uses data mining to optimize its supply chain by analyzing sales data, inventory levels, and logistics patterns. By leveraging advanced analytics, Walmart can predict demand fluctuations, ensuring products are stocked efficiently while minimizing overstock and shortages.
Through real-time data processing, Walmart enhances supplier coordination, reduces transportation costs, and improves delivery times. Machine learning algorithms also help the company anticipate seasonal trends, adjust pricing, and automate restocking. This data-driven approach not only boosts operational efficiency but also enhances customer satisfaction by ensuring product availability.
Amazon’s Customer Segmentation
Amazon uses data mining to segment its customers based on their purchasing behavior, demographics, and browsing history. This enables Amazon to offer targeted promotions, recommendations, and personalized marketing campaigns.
Procter & Gamble’s Customer Insight
Procter & Gamble uses data mining to gain insights into customer behavior, preferences, and needs. This enables P&G to develop targeted marketing campaigns, improve product development, and enhance customer satisfaction
Data Mining and Social Media
One of the most lucrative applications of data mining has been undertaken by social media companies. Platforms like Facebook, TikTok, Instagram, and X gather vast amounts of data about their users based on their online activities.
That data can be used to make inferences about user preferences, allowing advertisers to target their messages to people most likely to respond positively.
However, social media data mining has become controversial, with reports exposing how intrusive it can be. Users may accept terms and conditions without fully understanding how their personal information is collected and sold.
Example: Facebook-Cambridge Analytica Scandal
A major cautionary example of social media data mining is the Facebook-Cambridge Analytica scandal.
During the 2010s, the British consulting firm Cambridge Analytica collected personal data from millions of Facebook users.
This data was analyzed and allegedly used in the 2016 U.S. presidential campaigns of Ted Cruz and Donald Trump.
It was also suspected of influencing other events, such as the Brexit referendum.
Facebook later paid $100 million in penalties for misleading investors about its consumer data practices.
This scandal highlighted ethical concerns around user privacy, transparency, and the potential misuse of data for political influence.
The Role of AI and Automation in Data Mining
ML algorithms and artificial intelligence (AI) streamline data analysis.
Automation speeds up processes like anomaly detection, data classification, and predictive modeling.
Integration with Apache Spark and other data visualization tools enhances usability and accessibility.
Challenges in Data Mining
Data Privacy & Security – Ensuring compliance with regulations like GDPR.
Scalability Issues – Handling vast amounts of data efficiently.
Data Quality – Addressing biases and inconsistencies in datasets.
Future of Data Mining
Advances in AI and deep learning will further automate and refine data mining processes.
Growing adoption in industries like IoT, blockchain, and autonomous systems.
Ethical AI practices will shape the responsible use of data mining.
A major event shaping the future of data mining is the Data Science Next Conference 2025, scheduled for May 7–9 at the Sheraton Amsterdam Airport Hotel. Industry leaders, AI researchers, and data professionals will gather to discuss the latest trends in machine learning, big data analytics, and ethical AI. Sessions will cover automation, visualization tools like Apache Spark, and real-world applications in cybersecurity, finance, and precision agriculture.
Conclusion
Data mining is a powerful tool that continues to evolve with AI and big data. While challenges exist, automation and advanced analytics are making data mining more accessible and efficient. Organizations leveraging data mining effectively will gain a competitive edge in the digital age.