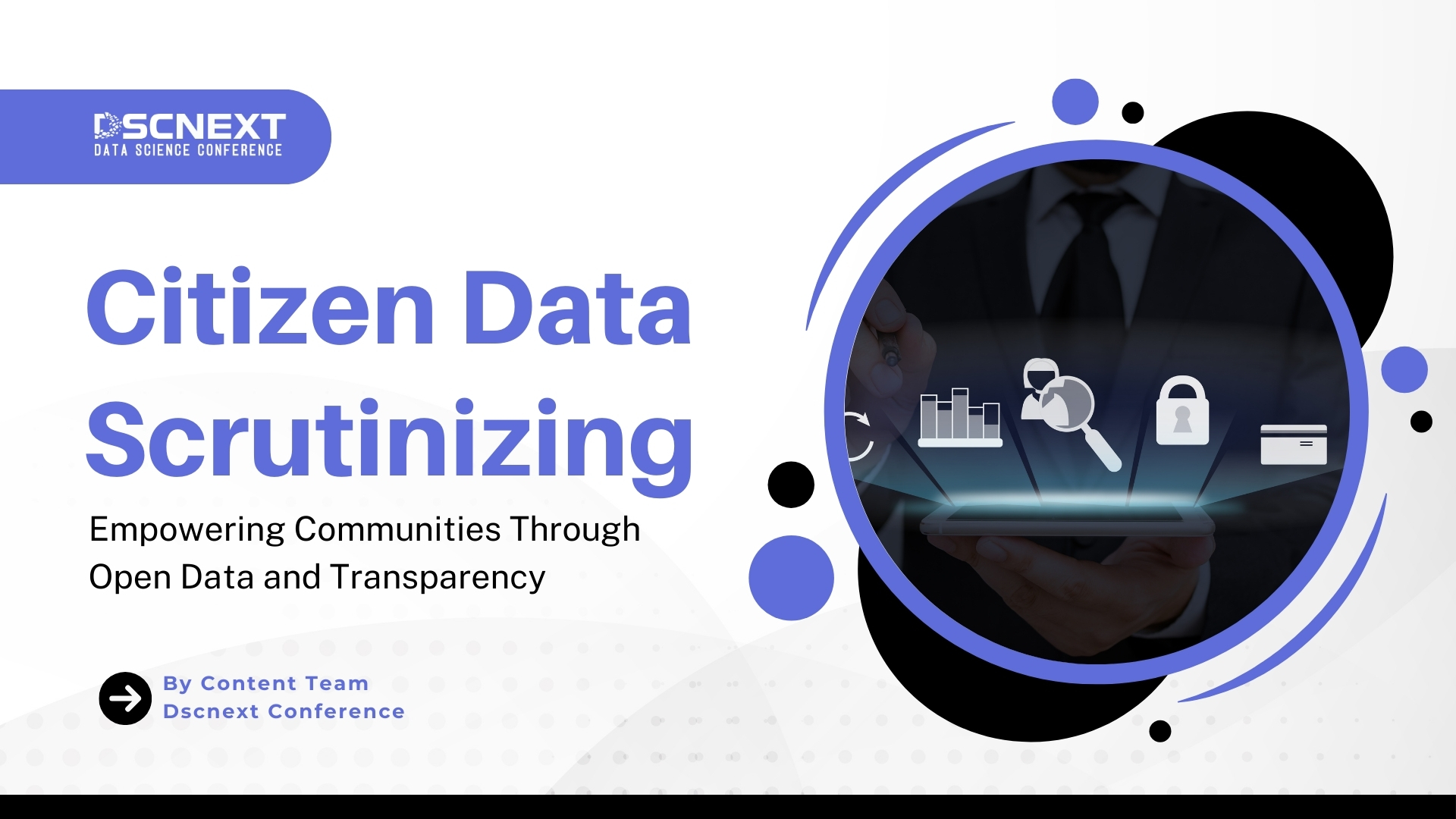
The rise of citizen data scrutinizing is a pivotal development in the ongoing democratization of data science. As data becomes increasingly integral to decision-making processes in society, the ability of everyday citizens to access, analyze, and interpret data is more critical than ever.
This movement empowers individuals and communities, traditionally excluded from the data-driven world, to engage meaningfully with data, hold institutions accountable, and contribute to societal change.
This write-up explores the rise of citizen data scrutinizing, who citizen data scientists are, and how they differ from professional data scientists. It also delves into the tools and methods used by these citizens, examines the societal impact, and discusses the challenges and risks associated with this trend.
1. Understanding Citizen Data Scrutinizing
Citizen data scrutinizing refers to the practice where non-expert individuals or groups independently examine, analyze, and draw conclusions from data. Unlike professional data scientists, these citizens are often motivated by personal, community, or civic concerns rather than corporate or institutional objectives.
The movement is fueled by the increasing availability of open data, advancements in user-friendly analytical tools, and a growing societal emphasis on transparency and accountability. In Flint, Michigan, citizens and local organizations used publicly available water quality data to expose elevated lead levels, leading to significant public health and policy responses. In India, initiatives like Open Budgets India have enabled citizens to scrutinize government budget data, enhancing transparency and fostering informed public debate on government spending.
2. Who Are Citizen Data Scientists?
Citizen data scientists are individuals who, despite not having formal training in data science, engage in data analysis using accessible tools and platforms. They differ from professional data scientists in several key ways:
– Motivation: Citizen data scientists are often driven by personal or community interests, such as environmental concerns, public health, or social justice. In contrast, professional data scientists typically work within organizations to solve specific business problems.
– Training and Expertise: While data scientists usually have formal training in fields like statistics, computer science, or mathematics, citizen data scientists rely on self-taught skills, online courses, or community knowledge.
– Scope of Work: Citizen data scientists often focus on specific, localized issues, while professional data scientists may work on broader, more complex projects requiring advanced methodologies.
Despite these differences, citizen data scientists play a crucial role in democratizing data science by bridging the gap between data-driven insights and the public, often raising awareness and driving action on critical issues.
3. Democratizing Data Science
The democratization of data science refers to making data analysis accessible to everyone, not just experts. This movement has gained momentum due to several factors:
Open Data Initiatives:
– Government Open Data: Platforms like the UK’s data.gov.uk and the European Union’s open data portal provide access to vast amounts of public sector data, enabling citizens to analyze everything from environmental quality to government spending.
– Corporate Transparency: Companies are increasingly sharing data with the public, particularly in sectors like energy, finance, and transportation. For instance, Google’s COVID-19 mobility reports provided valuable insights into how public movement patterns changed during the pandemic.
User-Friendly Tools:
– Data Visualization: Tools like Tableau, Google Data Studio, and Power BI have made data visualization accessible to non-experts, allowing them to create meaningful visual representations of complex datasets.
– Coding Platforms: For those interested in deeper analysis, platforms like Jupyter Notebooks and open-source libraries in Python (e.g., Pandas, Matplotlib) offer powerful but accessible environments for data manipulation and visualization.
– No-Code/Low-Code Platforms: Tools like KNIME and Alteryx allow users to perform data analysis and build predictive models without needing to write code, further lowering the barrier to entry.
Education and Training:
– Online Courses: Platforms such as Coursera, edX, and Khan Academy offer courses on data science, data visualization, and statistical analysis that are accessible to the general public.
– Community Initiatives: Hackathons, data meetups, and community workshops provide opportunities for citizens to learn and collaborate on data projects. For example, DataKind connects data scientists with NGOs to work on data-driven solutions for social good.
4. Tools and Methods Used in Citizen Data Scrutinizing
Citizen data scientists use a variety of tools and methods to analyze and interpret data:
Data Collection and Access:
– APIs and Web Scraping: Citizens can use tools like Python’s BeautifulSoup or Scrapy to collect data from websites. APIs provided by governments, corporations, or NGOs allow direct access to datasets.
– Open Data Portals: Websites like Kaggle and Data.gov provide easy access to datasets across various domains, ranging from climate data to financial statistics.
Data Analysis:
– Spreadsheet Software: Tools like Microsoft Excel and Google Sheets remain popular for basic data analysis and visualization due to their accessibility and ease of use.
– Data Cleaning Tools: OpenRefine is a powerful tool for cleaning messy data, allowing users to transform data from one format to another and explore large datasets.
– Statistical Analysis: For more advanced analysis, citizen data scientists might use R or Python, taking advantage of packages like NumPy for numerical analysis or SciPy for scientific computing.
Visualization and Communication:
– Mapping Tools: GIS software like QGIS or web-based platforms like Mapbox allow citizens to create maps that visualize spatial data, which is particularly useful in environmental and urban planning contexts.
– Storytelling Platforms: Websites like Flourish and Shorthand help citizens create interactive data stories, making it easier to communicate complex information in a compelling way.
5. Impact on Society
Citizen data scrutinizing has far-reaching effects on society:
Promoting Accountability:
– Government Transparency: By independently analyzing data, citizens can hold governments accountable. In the UK, the Transparency and Accountability Initiative has supported efforts to monitor public spending and government contracts.
– Corporate Responsibility: Consumers and advocacy groups are increasingly using data to hold companies accountable for their environmental and social impact. For example, ethical consumer platforms use company data to rate and review the sustainability practices of businesses.
Empowerment:
– Community Action: Local communities can use data to advocate for changes in their neighborhoods. For instance, community groups in Chicago have used crime data to lobby for better policing strategies and public safety measures.
– Social Justice: Data has become a tool for social justice movements, with activists using it to highlight inequalities and push for policy changes. For example, the Black Lives Matter movement has utilized data on police violence to support its calls for reform.
Driving Innovation:
– Citizen Science Projects: Initiatives like the Zooniverse platform enable citizens to contribute to scientific research by analyzing data in areas ranging from astronomy to biology.
– Public-Private Partnerships: Collaborations between citizens, governments, and businesses have led to innovative solutions to urban challenges. For example, the Smart Citizen Kit project in Barcelona has equipped citizens with sensors to collect data on air quality, noise, and temperature, influencing city planning.
6. Challenges and Risks
While the democratization of data science offers many benefits, it also presents challenges:
Data Literacy:
– Risk of Misinterpretation: Without proper training, citizens may misinterpret data, leading to incorrect conclusions or the spread of misinformation.
– Education Gap: Efforts to democratize data science must address the varying levels of data literacy among the population, ensuring that everyone has the opportunity to participate meaningfully.
Data Quality and Privacy
– Incomplete Data: Publicly available data can be incomplete or lack context, making it difficult for citizens to draw accurate conclusions.
– Privacy Concerns: The availability of large datasets raises privacy issues, particularly when data is not sufficiently anonymized.
Bias and Representation:
– Selection Bias: Citizen-led initiatives may suffer from selection bias, where the data scrutinized does not represent the entire population. This can lead to skewed insights and conclusions.
– Exclusion of Marginalized Voices: If certain groups are not included in the data collection or analysis process, their perspectives and needs may be overlooked.
7. The Future of Citizen Data Scrutinizing
As technology continues to evolve, and as more data becomes available, the role of citizen data scientists will likely expand. The future of this movement will depend on the continued development of tools that make data analysis accessible, as well as efforts to improve data literacy across the population.
Potential Developments:
– AI and Machine Learning: As AI becomes more integrated into data analysis tools, even non-experts will be able to leverage sophisticated techniques for data interpretation.
– Enhanced Collaboration: We might see more collaborations between citizen data scientists, professional data scientists, and institutions, leading to richer insights and more impactful outcomes.
– Global Reach: The movement will likely expand globally, with more citizens from different cultural and socio-economic backgrounds participating in data scrutinizing efforts, bringing diverse perspectives to global issues.
In summary, the rise of citizen data scrutinizing is an essential component of the democratization of data science. It empowers individuals and communities, promotes transparency, and drives innovation. However, it also presents challenges that must be addressed to ensure data is used responsibly and effectively.
As data science continues to evolve, its impact on supply chain management will only grow, making it an essential tool for businesses aiming to stay competitive in a rapidly changing global market. The future of data science is bright, with AI at its core, driving the next wave of technological innovation.
Companies that invest in data science will be better positioned to navigate the complexities of the modern world, make informed decisions, and achieve long-term success. Embark on a groundbreaking journey at the inaugural Data Science Next Conference May 7-9 2025, in Amsterdam by NBM, where pioneers and visionaries will gather to chart new territories in data science.
As a debut event, this conference offers an unparalleled opportunity to be among the first to explore fresh perspectives, engage with cutting-edge methodologies, and contribute to shaping the future of the field. Designed for those eager to push boundaries and spark innovation, this event promises to ignite your curiosity and provide the foundational insights needed to navigate the evolving landscape of data science. Join us as we set the stage for the next era of data-driven innovation.