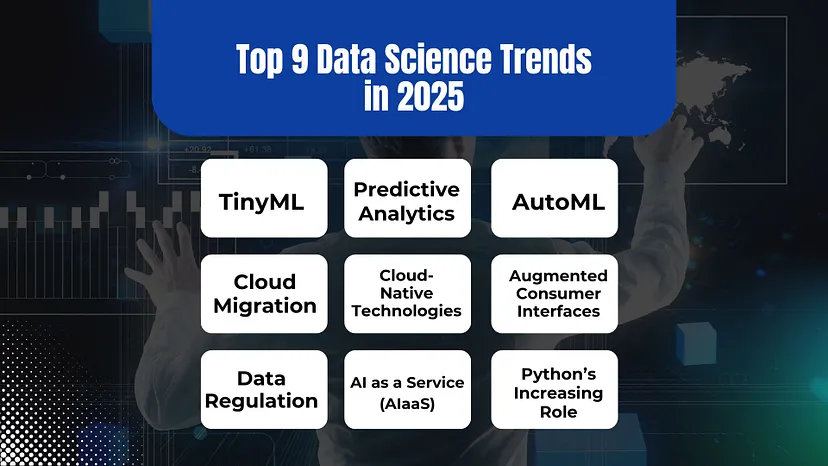
In 2025, data will be more prevalent than ever, with rapid growth reshaping industries. Data science, a combination of statistics, computer science, and domain expertise, will continue evolving, empowering businesses with smarter decision-making tools.
In this article, we explore the growth of data science technology and the top 9 data science trends to watch for in 2025, highlighting advancements in healthcare, banking, and beyond.
Data Science Technology Growth
The data science market is booming. Projections show that the market will reach USD 322.9 billion by 2026, driven by the increasing need to manage vast volumes of data for decision-making. By 2025, it’s estimated that 181 zettabytes of data will exist globally; far more than what was produced just a decade ago, which was merely nine zettabytes. As such, the demand for data science will only intensify.
Big data analytics is already widespread in sectors like healthcare, where 56% of healthcare centers have adopted predictive analytics. The growth of data science tools is essential for handling the data deluge and providing actionable insights.
9 Emerging Data Science Trends for 2025
Here are the top 9 data science trends that will shape 2025:
1. TinyML
TinyML focuses on deploying machine learning models on small, low-power devices like sensors and IoT gadgets. By enabling edge computing, it allows real-time data processing near the source, enhancing efficiency.
Example: Smart irrigation systems in agriculture use TinyML to monitor soil moisture and adjust water distribution, reducing waste and improving crop yields.
2. Predictive Analytics
Predictive analytics, powered by machine learning and statistical models, remains crucial in 2025. Enhanced cloud computing and processing tools will drive its adoption in areas like marketing, risk management, and supply chain forecasting.
Example: Retailers like Amazon leverage predictive analytics to forecast demand and manage inventory, ensuring seamless operations.
3. AutoML
Automated Machine Learning (AutoML) simplifies model building, making machine learning accessible to non-experts. This democratization boosts productivity across industries by allowing businesses to apply AI without extensive technical expertise.
Example: Google’s AutoML tools enable startups to create AI models for customer service chatbots without requiring in-house AI teams.
4. Cloud Migration
Cloud migration focuses on transferring data, applications, and systems from on-premises infrastructure to the cloud. This transition improves scalability, reduces costs, and supports real-time data processing. By 2025, cloud migration will continue to dominate as organizations embrace flexible and remote-friendly infrastructure.
Example: Netflix’s migration to AWS enabled seamless management of its vast content library and enhanced user data processing.
5. Cloud-Native Technologies
Cloud-native technologies, including containerization and microservices, optimize how businesses build and run scalable applications. These technologies are essential for accelerating innovation and ensuring seamless deployment cycles in dynamic environments.
Example: Shopify’suse of Kubernetes for deploying microservices ensures smooth operations during high-traffic sales events like Black Friday.
6. Augmented Consumer Interfaces
AI-powered interfaces will redefine user experiences in 2025. With IoT, VR, and AR integrations, businesses will create more personalized and engaging platforms for consumers.
Example: IKEA’s AR app allows customers to visualize how furniture fits in their homes before purchasing, enhancing the shopping experience.
7. Data Regulation
Data privacy and security will dominate in 2025, shaped by regulations like the EU Digital Services Act and AI Act. These policies will significantly impact data handling, especially in sensitive sectors like healthcare and finance.
Example: Healthcare providers in Europe ensure compliance with GDPR to protect patient data, avoiding hefty fines.
8. AI as a Service (AIaaS)
AIaaS will make AI solutions more accessible to businesses by eliminating hefty upfront investments. Tools like OpenAI’s GPT models and Google’s AI services will drive widespread adoption.
Example: Small businesses use OpenAI’s GPT to create customer support systems, saving costs on software development.
9. Python’s Increasing Role
Python remains the most popular language for data science and machine learning due to its simplicity and vast libraries like Pandas and Scikit-learn.Its versatility makes it indispensable for fields ranging from bioinformatics to game development.
Example: Tesla uses Python for developing autonomous vehicle algorithms, demonstrating its significance in cutting-edge technology.
Challenges in Data Science
Data science, while a rapidly evolving and essential field, faces several challenges that can hinder its effectiveness and implementation across various industries. These challenges stem from data quality issues, talent shortages, resistance to change, and the necessity for clear governance and transparency. For instance, a leading e-commerce company faced a $10M revenue loss due to flawed customer data, underscoring the criticality of robust data governance.
Data Quality and Governance
One of the most pressing challenges in data science is ensuring data quality. Organizations often deal with data that is inconsistent, inaccurate, or poorly formatted, which can lead to distorted insights and flawed decision-making
Implementing professional-grade data governance practices is crucial for monitoring and maintaining data integrity. Organizations may need to invest in data cleaning and preprocessing techniques to enhance data quality and optimize decision-making processes
Case Study
Data Science in Finance
Data science has emerged as a pivotal force in the finance sector, facilitating a shift towards customer-centric models. For example, algorithmic trading leverages advanced algorithms to execute trades at high speeds, significantly improving market efficiency and profitability for financial institutions
Real-world examples include hedge funds like Renaissance Technologies, which utilize sophisticated algorithms to identify market inefficiencies
Moreover, robo-advisors such as Betterment and Wealthfront employ data algorithms to provide personalized investment advice, democratizing access to financial planning
In terms of risk management, data science employs predictive analytics to identify potential threats, fundamentally changing how institutions approach risk
The growing integration of machine learning and deep learning techniques is set to transform quantitative finance further, enabling more complex financial analysis and decision-making processes
Conclusion
2025 will be a defining year for data science as it transforms industries and enhances decision-making processes.
From TinyML to AIaaS, the tools and technologies outlined here will drive innovation and efficiency across sectors.
Stay ahead of the curve by exploring these trends at the Data Science Conference 2025, where the future of data-driven innovation takes center stage.
The Data Science Conference 2025 will further spotlight these advancements, offering a global stage for showcasing the future of data-driven solutions.