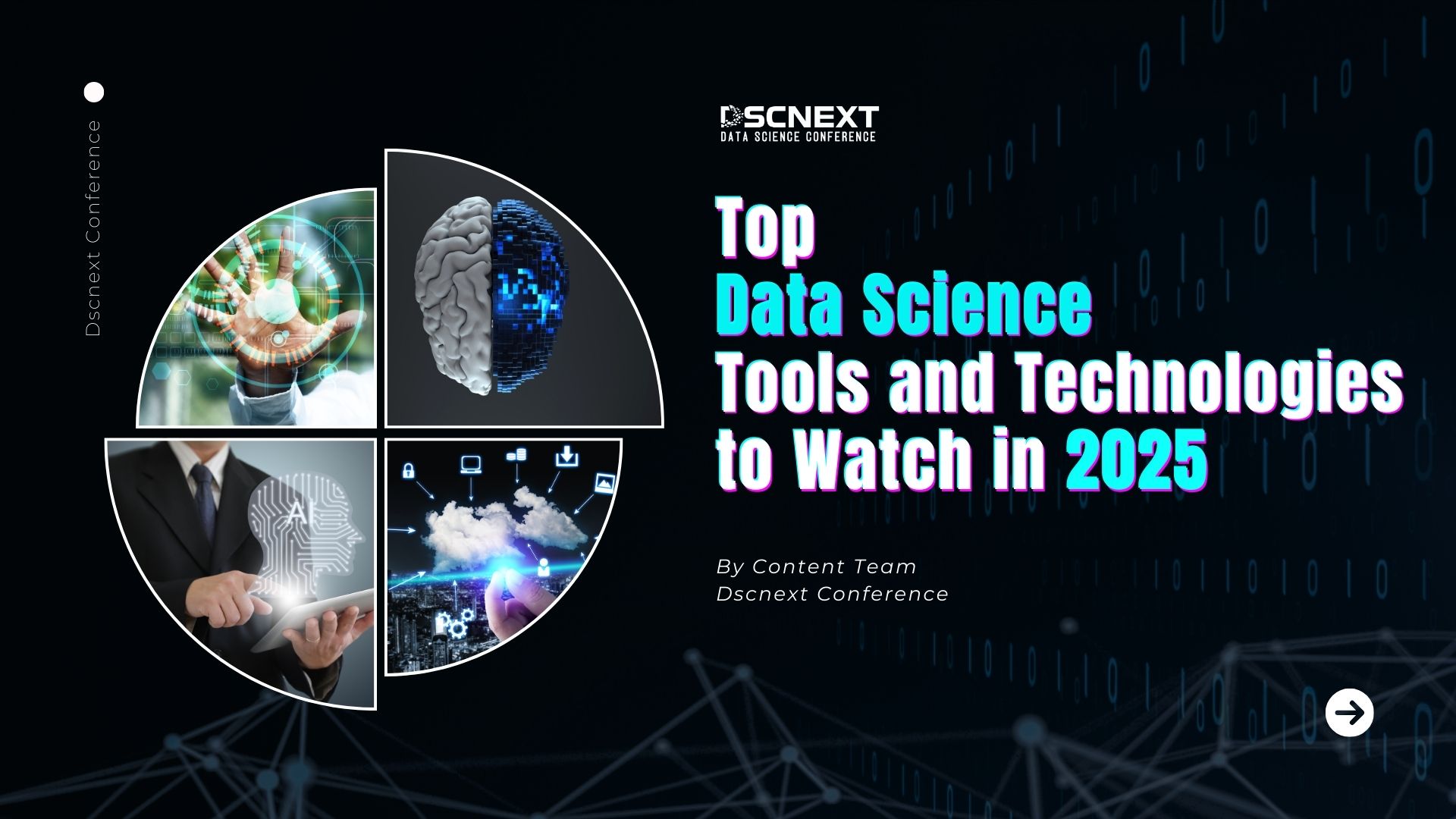
As the world becomes increasingly data-driven, the field of data science continues to evolve, driven by advancements in tools and technologies that offer greater capabilities for analyzing and leveraging data. With 2025 on the horizon, several tools and technologies are emerging as frontrunners in shaping the future of data science. Whether you’re a seasoned data scientist or an organization looking to harness data’s potential, these innovations are poised to make a significant impact.
1. AutoML: Democratizing Machine Learning
AutoML, or Automated Machine Learning, has revolutionized how data science is practiced by automating the end-to-end process of applying machine learning to real-world problems. In 2025, AutoML platforms like Google AutoML, DataRobot, and H2O.ai are expected to further democratize data science by enabling non-experts to build complex machine learning models with minimal coding. These tools streamline tasks such as data preprocessing, feature engineering, and model selection, making AI more accessible across industries.
2. Federated Learning: Privacy-First Data Analysis
Privacy concerns continue to be a significant barrier in data science, particularly in industries like healthcare and finance. Federated learning offers a solution by allowing models to be trained across multiple devices or organizations without transferring raw data to a central server. By 2025, technologies like TensorFlow Federated and PySyft are likely to gain traction, allowing organizations to collaborate on machine learning projects while maintaining data privacy.
3. Graph Analytics: Unlocking Complex Relationships
Graphs are an excellent way to represent complex relationships, such as social networks or supply chains. Graph analytics tools like Neo4j, TigerGraph, and Amazon Neptune are expected to see increased use in 2025 as organizations leverage them for insights into relational data. These tools are particularly valuable for fraud detection, recommendation systems, and knowledge graphs, where the ability to understand connections between entities is crucial.
4. MLOps: Streamlining Model Lifecycle Management
As machine learning models move from research to production environments, the need for streamlined operations has become clear. MLOps (Machine Learning Operations) helps manage the lifecycle of ML models, from development to deployment and monitoring.
By 2025, platforms like Kubeflow, MLflow, and Seldon will continue to advance, enabling organizations to scale their AI initiatives more effectively. These tools integrate data pipelines, automate deployment, and monitor performance, ensuring that models remain accurate and effective over time.
5. Quantum Computing: The Next Frontier
Although still in its early stages, quantum computing is anticipated to revolutionize data science by solving problems that are intractable for classical computers. Tools like IBM’s Qiskit, Google Cirq, and Microsoft’s Azure Quantum are laying the foundation for this transformative technology. By 2025, quantum computing could begin to offer real-world applications in optimization problems, cryptography, and complex simulations, though widespread commercial use may still be years away.
6. Natural Language Processing (NLP) Advancements
Natural Language Processing (NLP) continues to evolve, with new advancements in transformer models like GPT-4 and BERT leading the charge. By 2025, expect even more powerful tools for text analysis, sentiment analysis, and conversational AI. Tools like Hugging Face’s Transformers, spaCy, and OpenAI’s API are likely to become even more sophisticated, enabling more accurate language models that can perform tasks like summarizing large datasets, automating report generation, or even providing customer support.
7. Data Visualization Tools: More Intuitive and Interactive
Data visualization remains a critical aspect of data science, helping teams and stakeholders understand complex data insights. By 2025, we can expect more advanced and interactive visualization tools like Tableau, Power BI, and Plotly to enhance user experiences with real-time dashboards, AI-driven insights, and augmented analytics. These tools will help transform raw data into actionable insights, allowing users to make data-driven decisions quickly and effectively.
8. Edge Computing: Real-Time Analytics at the Source
As IoT devices proliferate, edge computing is becoming essential for real-time data analytics closer to the data source. In 2025, edge computing platforms like AWS IoT Greengrass, Azure IoT Edge, and NVIDIA’s Edge AI will allow data scientists to deploy models directly onto devices, reducing latency and improving performance. This is especially important for industries like manufacturing, transportation, and healthcare, where real-time decisions can make a significant difference.
9. Augmented Analytics: AI-Powered Insights
Augmented analytics uses machine learning and AI to automate insights, making it easier for business users to interact with data without needing a deep understanding of data science. Tools like ThoughtSpot, Qlik Sense, and SAP Analytics Cloud are expected to become increasingly popular by 2025, offering AI-driven analytics and enabling users to ask questions in natural language and receive data-driven insights. This shift empowers more people within an organization to leverage data effectively.
10. Big Data Processing Tools: Scalability and Efficiency
With the explosion of data volumes, big data processing tools remain essential for managing and analyzing massive datasets. In 2025, technologies like Apache Spark, Databricks, and Google BigQuery will continue to dominate the landscape, offering scalable, cloud-based solutions for distributed computing. These tools help data scientists process vast amounts of data quickly, enabling faster insights and more efficient workflows.
Conclusion
As we look toward 2025, the future of data science is bright, with advancements in tools and technologies set to unlock new possibilities. From quantum computing to federated learning and augmented analytics, these innovations will empower organizations to harness data more effectively, driving decision-making and business growth. Staying updated on these trends will be critical for data professionals and organizations aiming to remain competitive in the evolving digital landscape.
By embracing these emerging tools, data scientists can stay at the forefront of innovation, ready to tackle the complex challenges and opportunities that lie ahead.